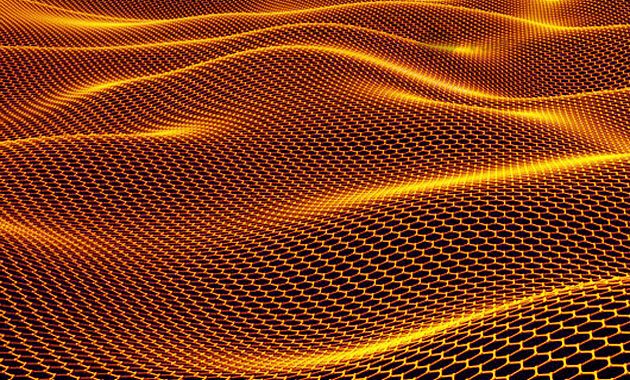
Researchers from Skoltech and their colleagues from Germany and the US have studied the properties and behavior of a palladium-copper alloy under changing temperatures and hydrogen concentrations, with highly relevant implications of this research for catalyst design. The paper was published in the Journal of Applied Physics.
Transition metal-alloy materials can have catalytic properties and are widely used in facilitating various chemical reactions such as CO2 hydrogenation, a process that turns carbon dioxide into methanol. Using an alloy of a more expensive reactive element with another one that is cheaper and more inert makes these catalysts highly efficient. One example of such a catalyst would be an alloy of palladium (Pd) and copper (Cu), where isolated atoms of Pd are positioned in the Cu lattice.
Zhong-Kang Han, Debalaya Sarker and Sergey Levchenko of the Skoltech Center for Energy Science and Technology (CEST) and their colleagues modelled the properties of a Pd/Cu alloy, using a machine-learning model to predict the distribution of Pd atoms on a Cu surface as a function of hydrogen partial pressure and temperature. “Only Pd atoms at the surface provide catalytically active sites. Therefore, it is important to know how many of these atoms can be found at the surface at relevant temperatures and hydrogen partial pressures,” Levchenko says.
He says that evaluating the energies of many atomic configurations of Pd within the Cu lattice requires a lot of computational resources, so the researchers chose a surrogate cluster expansion model that is easier to handle. “This model allows us to evaluate energy of millions of configurations in seconds. In this study we had a system that is more complex than the ones typically studied using cluster expansion: a surface of an alloy where the stability of various atomic configurations is influenced by adsorbates from the gas phase. Therefore, we applied the machine-learning approach based on compressed sensing (a method widely used to compress images) to develop a very accurate and predictive surrogate model,” Levchenko notes.
The team found that hydrogen adsorption indeed has a strong effect on the concentration of Pd atoms in the top layer of Cu (111) surface. “While at low hydrogen partial pressures and higher temperatures Pd prefers to stay at the surface, at higher pressures and lower temperatures hydrogen adsorption drives Pd away from the surface,” Levchenko explains.
The authors hope that their findings can open the door for designing metal alloys with better catalytic properties by taking into account dynamic changes in the composition and structure of materials at realistic operational conditions.
Other organizations involved in this research include Humboldt-Universität zu Berlin and the University of Pittsburg.
Read the most up to date Fuel Cell and Hydrogen Industry news at FuelCellsWorks